ML Advanced Methods #2 (אירוע)
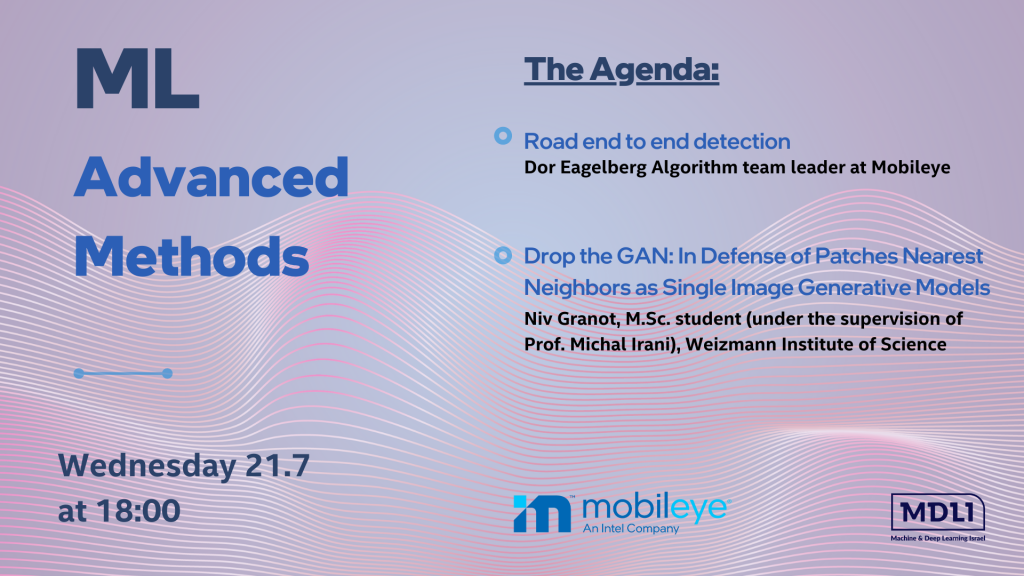
אני שמח להזמין אתכם לאירוע השני שלנו בסדרת ML Advanced Methods שנעשים בשיתוף עם Mobileye. בכל אירוע מסוג זה, נסקור מספר עבודות בנושאים מתקדמים בתחום ה-ML ונציג פרקטיקות נפוצות לשימוש במודלים שונים. הפעם יש לנו שני דוברים שידברו על נושאים מרתקים במיוחד בתחום הראייה הממוחשבת -פרטים נוספים בהמשך הכתבה. האירוע יתקיים ב-21.7 בשעה 18:00.
ההרשמה מתבצעת דרך הלינק הזה.
הרצאה ראשונה:
הרצאה שנייה:
Full name: Niv Granot,
Title: M.Sc. student (under the supervision of Prof. Michal Irani), Weizmann Institute of Science
Lecture Title: Drop the GAN: In Defense of Patches Nearest Neighbors as Single Image Generative Models
Abstract:
Single image generative models perform synthesis and manipulation tasks by capturing the distribution of patches within a single image. The classical (pre Deep Learning) prevailing approaches for these tasks are based on an optimization process that maximizes patch similarity between the input and generated output. Recently, however, Single Image GANs were introduced both as a superior solution for such manipulation tasks, but also for remarkable novel generative tasks. Despite their impressiveness, single image GANs require long training time (usually hours) for each image and each task. They often suffer from artifacts and are prone to optimization issues such as mode collapse. We show that all of these tasks can be performed without any training, within several seconds, in a unified, surprisingly simple framework. The “good-old” patch-based methods are revisited and casted into a novel optimization-free framework. This allows generating random novel images better and much faster than GANs. We further demonstrate a wide range of applications, such as image editing and reshuffling, retargeting to different sizes, structural analogies, image collage and a newly introduced task of conditional inpainting. Not only is our method faster (×103-×104 than a GAN), it produces superior results (confirmed by quantitative and qualitative evaluation), less artifacts and more realistic global structure than any of the previous approaches (whether GAN-based or classical patch-based).